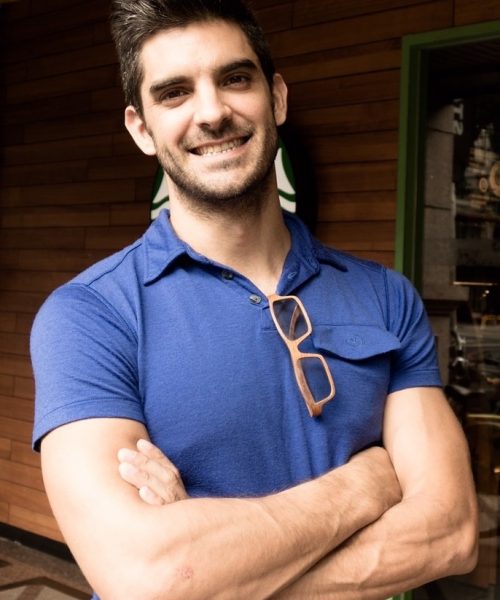
Stefano Rini
Associate Professor Department of ECE
National Chao Tung University
Title:
Communication-Efficient Federated Learning: Challenges, Techniques, and Insights from M22
Time: Wednesday, May 3rd 2023, 11:30 – 12:10
Abstract
Federated Learning (FL) has emerged as a powerful approach to training large models on distributed datasets, offering advantages in terms of data locality, scalability, and privacy. However, practical implementation of FL faces significant challenges, primarily due to the communication constraints between remote learners and the Parameter Server (PS). In this talk, we will provide a comprehensive survey of the current state of communication-efficient federated learning, exploring the various techniques and methodologies that have been proposed to address these challenges.
We will first discuss the practical issues arising from distributed training, focusing on the communication bottleneck between remote learners and the PS. Next, we will delve into the two main classes of gradient compression algorithms: gradient sparsification and gradient quantization. Furthermore, we will explore the role of dimensionality reduction algorithms and error feedback mechanisms in improving training performance.
Our contribution, the M22 algorithm, will be presented in the context of these broader developments. M22, a rate-distortion inspired approach to gradient compression, leverages an M-magnitude weighted L2 distortion measure and 2 degrees of freedom distribution fitting to achieve efficient compression in FL scenarios.
Biography:
Stefano Rini is currently an Associate Professor in the Department of Electrical and Computer Engineering at National Yang-Ming Chiao-Tung University (NYCU) in Taiwan. He received his Ph.D., M.S. in Electrical and Computer Engineering, and M.S. in Statistics from the University of Illinois at Chicago (UIC), where he maintained a perfect 4.0 GPA. Stefano also holds a B.A. in Computer Science from Politecnico di Milano, where he graduated with high honors.
His research interests are diverse and include machine learning and communication, with a particular focus on decentralized learning, federated learning, and distributed computation. He is also interested in data compression for deep learning, specifically in gradient compression, model compression, and approximate computation. Stefano explores information theory and machine learning theory, where he examines topics such as gradient compression, the straggler problem, and rate-distortion theory for DNN training.
Additionally, Stefano has delved into compressed sensing and its applications, working on projects related to compressed sensing for multiple access, direction of arrival estimation, and matrix completion for signal estimation. His work in medical imaging encompasses electrical impedance tomography and ultrasound imaging. Stefano has also researched information processing in the human auditory system, focusing on the design of cochlear implant stimulation and speech enhancement through auditory nerve fiber simulation.
Throughout his career, Stefano has held several prestigious positions, including post-doctoral positions at Stanford University and Technische Universität München (TUM), where he worked on cutting-edge research projects in the aforementioned areas.